This article will review two powerful and timesaving methods available from Pandas and Numpy that can be applied to problems that frequently arise in Data Analysis. These techniques are especially applicable to what is sometimes called ‘binning’ -transforming continuous variables into categorical variables.
The two methods are np.select() and pd.cut(). I call these Pandas tricks, but some of the actual implementation is taken from Numpy and applied to a Pandas container.
np.select()
Most Pandas programmers know that leveraging C-based Numpy vectorizations is typically faster than looping through containers. A vectorized approach is usually simpler and more elegant as well–though it may take some effort to not think in terms of loops. Let’s take an example of assigning qualitative severity to a list of numerical vulnerability scores using the First.org CVSS v3 mapping:
Rating | CVSSv3 Score |
---|---|
None | 0.0 |
Low | 0.1 – 3.9 |
Medium | 4.0 – 6.9 |
High | 7.0 – 8.9 |
Critical | 9.0 – 10.0 |
Suppose you have a large DataFrame of scores and want to quickly add a qualitative severity column. the np.select() method is a fast and flexible approach for handling this transformation in a vectorized manner. We can begin by making up some fictional CVEs as our starting dataset
import numpy as np
import pandas as pd
df = pd.DataFrame({"CVE":["CVE-2030-"+ str(x) for x in range(1,101)],"CVSSv3":(np.random.rand(100)*10).round(2)})
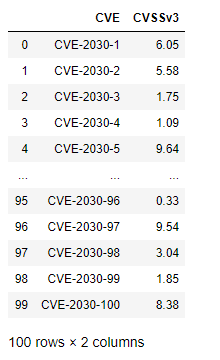
The above is a quick way to build a random DataFrame of made-up CVEs and scores for demonstration purposes. Using np.select() presents a slick vectorized approach to assign them the First.org qualitative label.
Begin by assembling a Python list of conditions based on the First.org labels, and place outcomes in separate Python list.
conditionals = [
df.CVSSv3 == 0,
(df.CVSSv3 >0) & (df.CVSSv3 <=3.95),
(df.CVSSv3 >3.95) & (df.CVSSv3 <=6.95),
(df.CVSSv3 >= 6.95) & (df.CVSSv3 <=8.95),
(df.CVSSv3 >= 8.95) & (df.CVSSv3 <= 10)
]
outcomes = ["None","Low","Medium","High","Critical"]
Using the Conditionals
At this point a new qualitative label can be applied to the dataset with one vectorized line of code that invokes the conditionals and labels created above:
df["Severity"] = np.select(conditionals,outcomes)
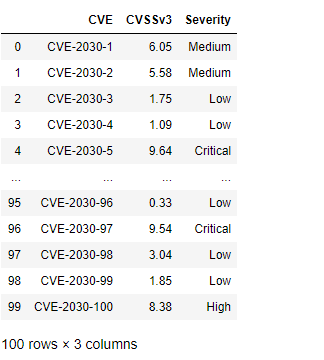
pd.cut()
An alternative approach, one that is arguably programmatically cleaner but perhaps less flexible, is the Pandas cut() method. There are lot of options for using pd.cut(), so make sure to take some time to review the official documentation to understand the available arguments.
One option is to set up a series of intervals using a Python list. It is important to remember that the intervals will, by default, be closed to the right and open to the left. Below is an initial attempt to pattern intervals after the First.org boundaries
bins = [0,0.01,3.95, 6.95, 8.95, 10]
Now that the boundaries are established, simply add them as arguments in a call to pd.cut()
df["Severity2"] = pd.cut(df.CVSSv3,bins, labels=["None","Low","Medium","High","Critical" ], retbins=False)
This results in the following
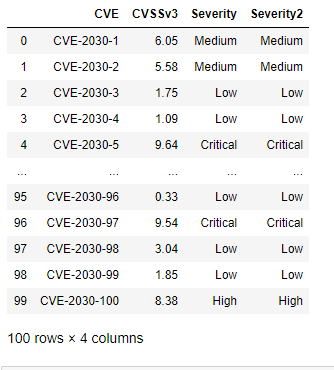
As always, it is prudent to do some testing: especially to test the boundary conditions. We can redo the array-building exercise while appending a few custom rows at the end using the following code:
np.random.seed(100)
df = pd.DataFrame({"CVE":["CVE-2030-"+ str(x) for x in range(1,101)],"CVSSv3":(np.random.rand(100)*10).round(2)})
df.loc[len(df.index)] = ['CVE-2030-101', 0]
df.loc[len(df.index)] = ['CVE-2030-102', 10]
df.loc[len(df.index)] = ['CVE-2030-103', 0.1]
df.loc[len(df.index)] = ['CVE-2030-104', 9.0]
df.loc[len(df.index)] = ['CVE-2030-105', 4.0]
df.loc[len(df.index)] = ['CVE-2030-106', 3.96]
df.tail(10)
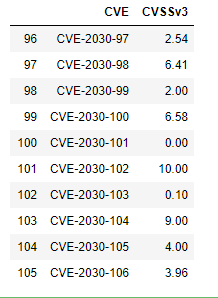
The df.loc[len(df.index)] simply locates the index value needed to add an additional row. Setting a random seed in line 1 above makes the DataFrame reproducible despite the values being randomly generated.
Now, if this were a unit test, then we would assert that CVE-2030-101 would have qualitative value ‘None’ and CVE-2030-106 would have qualitative value ‘Medium’. Note that for readability we can also improve the way we label the columns of the DataFrame to more readily identify the type of binner employed. Running the binner routines results in:
np.random.seed(100)
df = pd.DataFrame({"CVE":["CVE-2030-"+ str(x) for x in range(1,101)],"CVSSv3":(np.random.rand(100)*10).round(2)})
df.loc[len(df.index)] = ['CVE-2030-101', 0]
df.loc[len(df.index)] = ['CVE-2030-102', 10]
df.loc[len(df.index)] = ['CVE-2030-103', 0.1]
df.loc[len(df.index)] = ['CVE-2030-104', 9.0]
df.loc[len(df.index)] = ['CVE-2030-105', 4.0]
df.loc[len(df.index)] = ['CVE-2030-106', 3.96]
df["Severity pd.cut()"] = pd.cut(df.CVSSv3,bins, labels=["None","Low","Medium","High","Critical" ])
bins = [0,0.01,3.95, 6.95, 8.95, 10]
df["Severity np.select()"] = np.select(conditionals,outcomes)
df.tail(10)
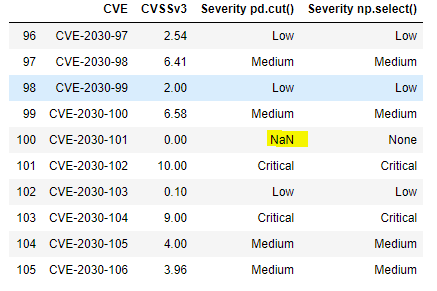
The boundary testing has turned up a problem: the pd.cut() doesn’t handle the value zero because the first interval – 0, 0.1 is open on the left. Such an interval is designated by math types as (0, 0.1], with the parenthesis indicating that zero is not contained in the interval. This bug is easily addressed–the interval can be started at -.99 rather than zero. Alternatively, one could use the method’s arguments to adjust the open-on-left, closed-on-right default behavior.
The pd.cut() bin intervals are adjusted as:
bins = [-.99,0.01,3.95, 6.95, 8.95, 10]
Running the entire code again looks like this:
np.random.seed(100)
df = pd.DataFrame({"CVE":["CVE-2030-"+ str(x) for x in range(1,101)],"CVSSv3":(np.random.rand(100)*10).round(2)})
df.loc[len(df.index)] = ['CVE-2030-101', 0]
df.loc[len(df.index)] = ['CVE-2030-102', 10]
df.loc[len(df.index)] = ['CVE-2030-103', 0.1]
df.loc[len(df.index)] = ['CVE-2030-104', 9.0]
df.loc[len(df.index)] = ['CVE-2030-105', 4.0]
df.loc[len(df.index)] = ['CVE-2030-106', 3.96]
bins = [-.99,0.01,3.95, 6.95, 8.95, 10]
df["Severity pd.cut()"] = pd.cut(df.CVSSv3,bins, labels=["None","Low","Medium","High","Critical" ])
df["Severity np.select()"] = np.select(conditionals,outcomes)
df.tail(10)
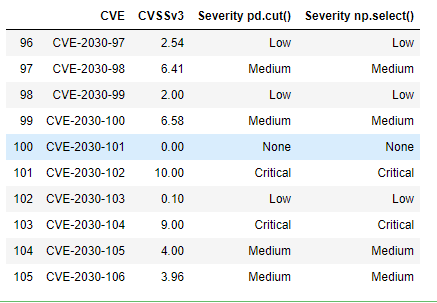
This is the result we were expecting.
Note one other interesting implementation detail; the CVSSv3 mapping from First.org uses precision = 1 and the code and data generation used here was done with precision = 2. That is a detail that may well have led to unexpected results. We could have adjusted the random generator to round to a single digit after the decimal, or simply reset the DataFrame using the round function:
df = df.round(1)